It may seem surprising to some, but ccNUMA has hit the mass market and will forcefully continue to do so by the end of 2008 with Intel’s Nehalem processor. And although ccNUMA bears the potential of vastly improved bandwidth scalability, many users and sysadmins meet it with ignorance. Alas, their ignorance is all too often vindicated by the fact that they are right – sometimes.
This is because the vast majority of parallel application codes uses MPI. If you run one MPI process per core and the kernel does an average job of maintaining affinity, you will benefit from ccNUMA without the hassle of paying attention to correct parallel page placement. The latter is mandatory with memory-bound OpenMP code and sometimes not easy to implement, in particular when the problem is not as regular as, say, a simple dense matrix-vector multiply.
However, even with MPI you can run into the ccNUMA trap when the system’s memory is filled with something else before your code starts to run. This could be, e.g., file system buffer cache. In order to pinpoint the problem, Michael has done an interesting test on one of our dual-socket (dual-LD) Opteron nodes and, for comparison, on a dual-socket Woodcrest system. The former is ccNUMA while the latter is UMA. The test performs the following steps in a loop:
- Drop the FS caches by “
echo 1 > /proc/sys/vm/drop_caches
“. Btw, this facility exists since Linux kernel 2.6.16. Earlier kernels may have similar features, but those are not “official”. This operation is equivalent to using the bcfree
command on an SGI Altix.
- Write a file of some size (increasing with iteration count) to the local disk. The maximum file size equals the amount of memory.
- sync the cache so that there are no flush operations in the background (but the cache is still filled).
- Run a vector triad benchmark with 4 MPI processes that fills exactly half of the node’s memory.
As the triad is purely bandwidth-bound, its performance depends crucially on the memory pages being allocated in the same locality domains as the processors that use them. However, the presence of a huge buffer cache prevents this:
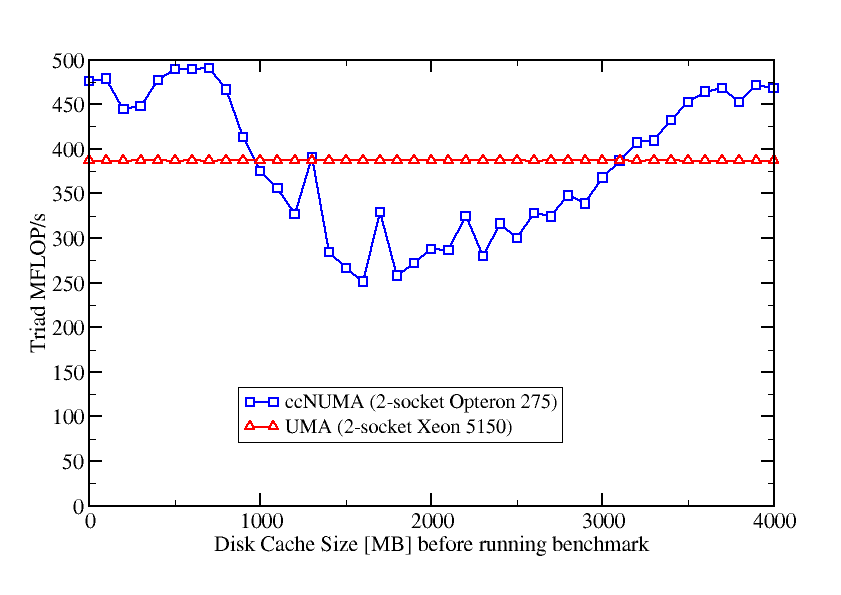
The code can get an aggregate performance of roughly 500 MFlop/s on the Opteron node and close to 400 MFlop/s on the Woodcrest. We see that performance is unharmed by the buffer cache on the UMA system, but there are huge fluctuations on the ccNUMA node. Minimum performance is reached if cache size is about 2 GB which is half of the installed memory. In this case the kernel has filled one LD with buffer cache and all MPI processes map their pages in the second LD. We end up with the well-known congestion problem – a single LD must service the bandwidth demands of all cores. With the file size growing even further the effect vanishes because if all memory is filled by FS cache, any user-allocated page will drop a cache page and access becomes local again.
Linux, by default, keeps the cache even if an application is forced to map memory pages from a foreign LD if there is nothing left in the local LD. You can prevent this, of course, by using appropriate calls from the libnuma library, but you have to know about this. Most users don’t.
On the admin’s side we see that it’s a good idea to drop the cache whenever a cluster’s compute node becomes free. Users can’t do this themselves because you have to be root. As a makeshift, however, a user can flush and drop all cache pages by running a “sweeper” code that allocates and touches all the node’s memory before the real application starts.
Summing up, users and admins must be aware of such effects. Imagine what happens with a job that uses hundreds of ccNUMA nodes on one of which there’s a huge FS cache…